The O word: do you really need an ontology? The Year of the Graph Newsletter: November / October 2019
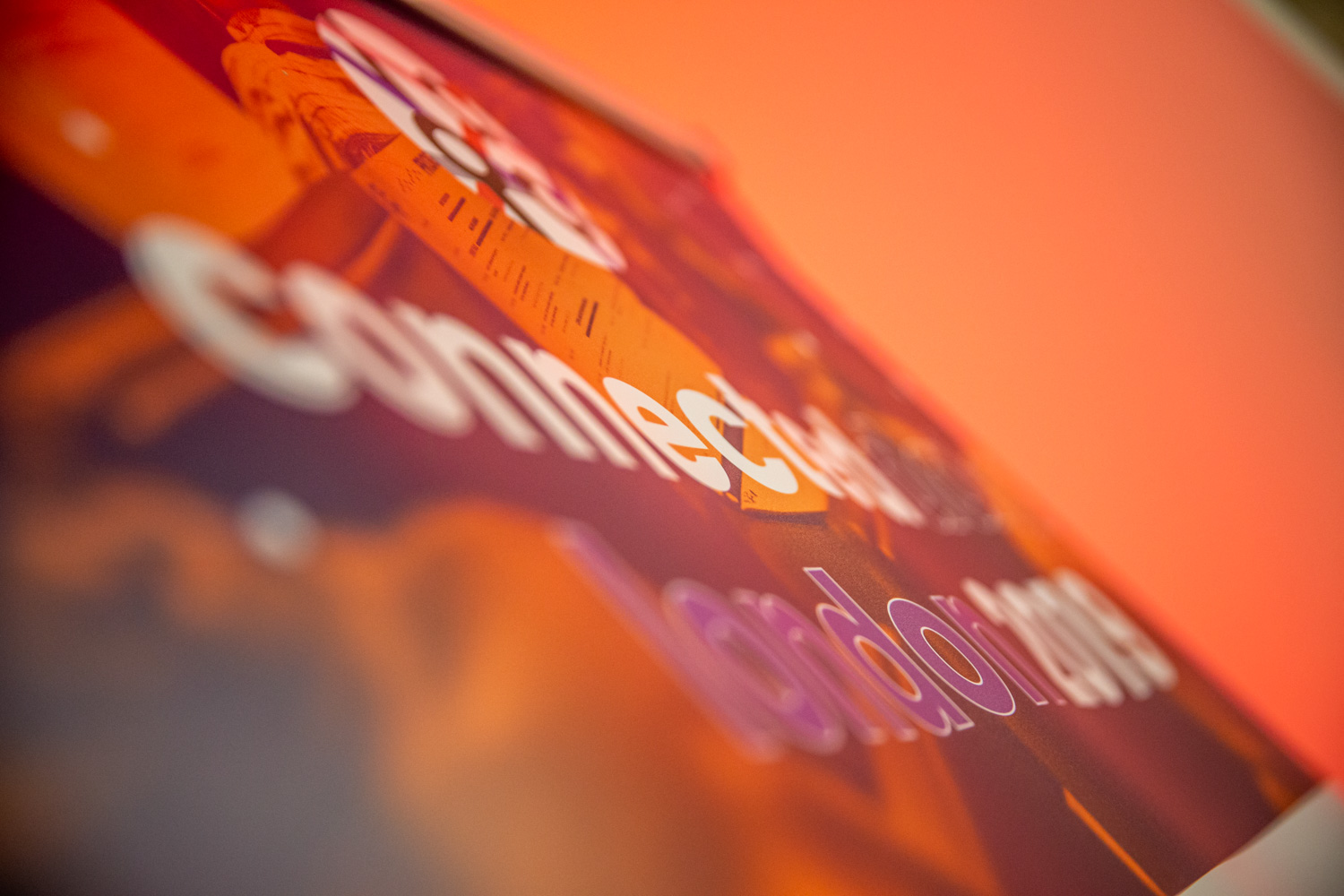
How do you manage your enterprise data in order to keep track of it and be able to build and operate useful applications? This is key question all data managements systems are trying to address, and knowledge graphs, graph databases and graph analytics are no different. What is different about knowledge graphs is that they […]
Read More →Knowledge Graphs and Natural Language Processing. The Year of the Graph Newsletter, July/August 2019
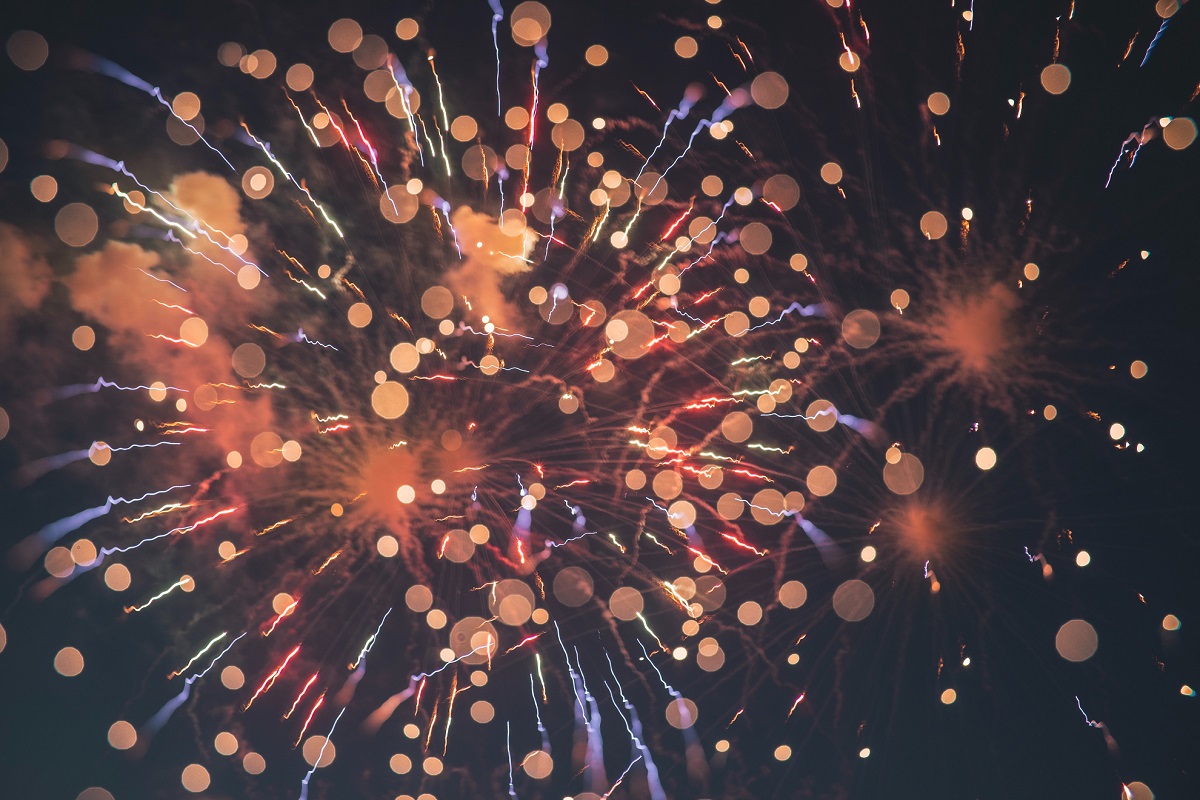
Pinterest gets with the knowledge graph program. Facebook releases a new dataset for conversational Reasoning over Knowledge Graphs. Connected Data London announces its own program, rich in leaders and innovators. And as always, new knowledge graph and graph database releases, research, use cases, and definitions. A double bill summertime newsletter edition, making your knowledge graph […]
Read More →Graphs in the cloud. The Year of the Graph Newsletter: April 2019

The first sign of convergence in the graph space is here. Graph databases continue to grow, expand, and make their way to the cloud, a number of open source frameworks for working with graphs has been released, and a slew of new interesting use cases. Read the full article on the Year of the Graph
Read More →Knowledge graphs, AI, and interoperability. The Year of the Graph Newsletter: February 2019

Knowledge graphs are spreading everywhere: from Airbnb and eBay to Alexa, and from using JSON-LD on the web for better SEO to leveraging taxonomy to define AI. Combining knowledge graphs and machine learning, benchmarking graph databases, and W3C initiative for interoperability shaping up. January 2019 has been a lively month in the graph landscape. Read […]
Read More →Graph gets funding. The Year of the Graph Newsletter Vol. 7, November 2018
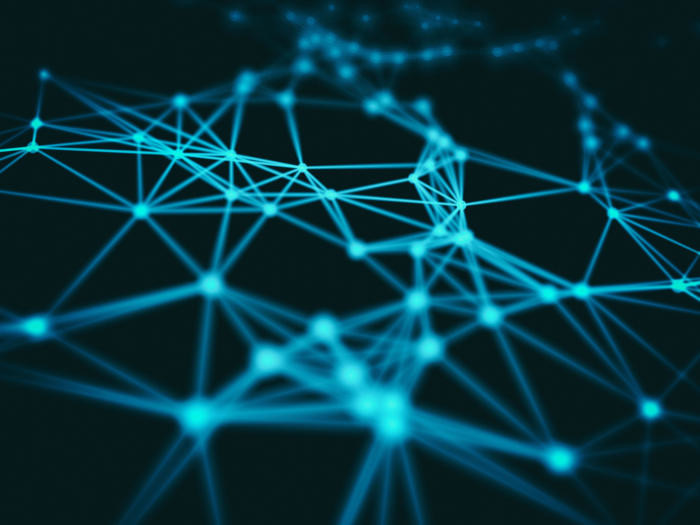
October 2018 was the busiest month in the busiest year in graph history, hence the longest Year of the Graph newsletter to date. Neo4j lands a massive funding round, Tinkerpop is moving forward, the most important knowledge graph research event with key industry presence, W3C organizing a Workshop on Web Standardization for Graph Data, and […]
Read More →Getting knowledge graph semantics and definitions right. The Year of the Graph Newsletter Vol. 6, October 2018
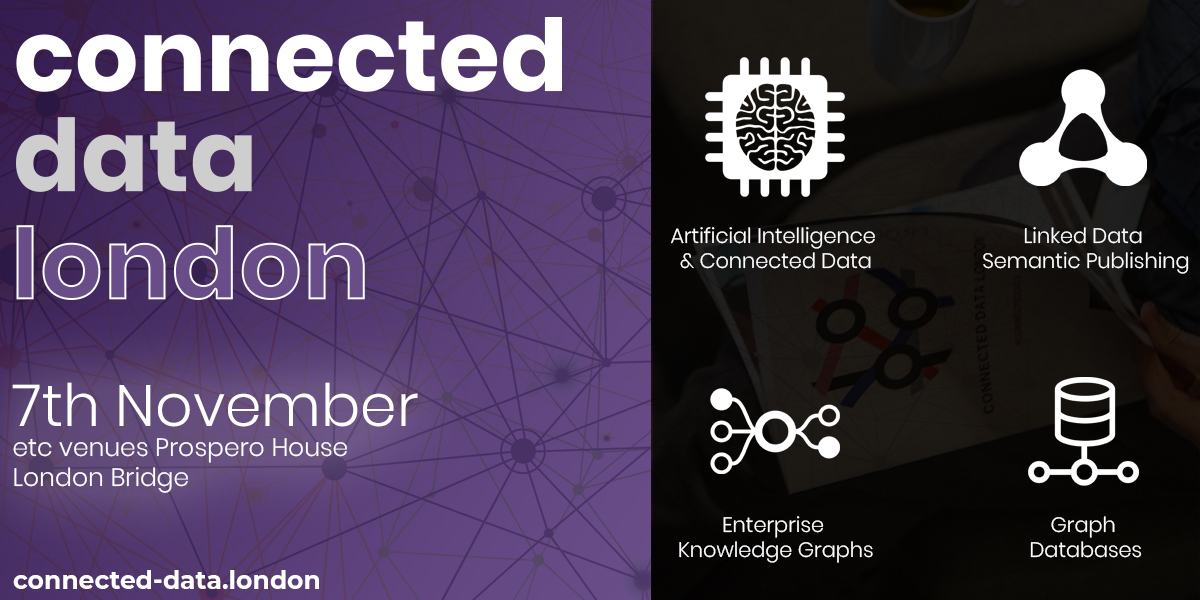
Getting knowledge graph semantics and definitions right, semantic web standards used in the real world, by Google no less, and ArangoDB, Azure CosmosDB, Neo4j and TigerGraph announcing new versions. By now you probably know that knowledge graphs are in Gartner’s Hype Cycle. But how does one actually define a knowledge graph? My take on ZDNet. […]
Read More →Knowledge graphs in Gartner’s hype cycle. The Year of the Graph Newsletter Vol. 5, September 2018

Knowledge graphs in Gartner’s hype cycle, machine learning extensions and visual tools for graph databases, Ethereum analytics with RDF, Using Gremlin with R, SPARQL, and Spring, graph database research wins best paper award in VLDB, and benchmarking AWS Neptune. Not bad for a typical summer vacation month such as August. This edition of the Year […]
Read More →On Graph query languages. The Year of the Graph Newsletter Vol. 3, June 2018

AWS Neptune goes GA, Microsoft Cosmos DB releases new features, the query language discussion heats up, TigerGraph announces free developer edition, building enterprise knowledge graphs in the real world with Zalando and Textkernel, and more. May has been another interesting month for the graph database world. How can data scientists use knowledge graphs? How, and […]
Read More →Here we go: The Year of the Graph Newsletter Vol. 1, April 2018
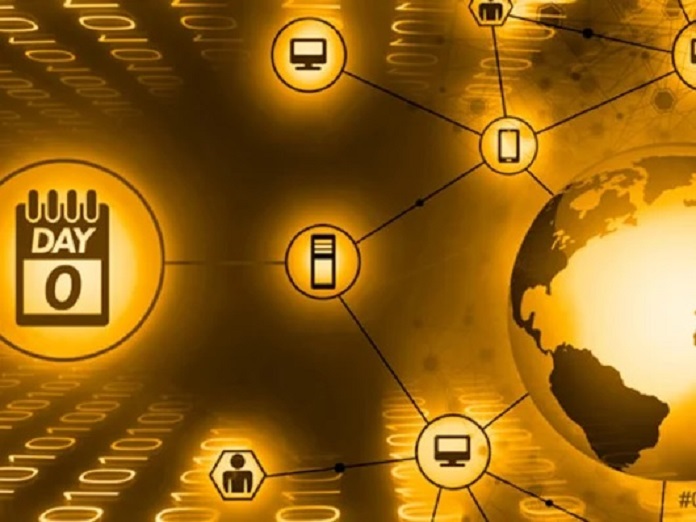
Graph databases are the hottest thing around right now. Whether you are just getting started, or you are in one of the 51% of organizations already using them, this is the place to get your news and analysis. The popularity of graph databases has gone through the roof almost overnight it seems. Everything points this way: […]
Read More →RDF on Hadoop and Schema on Read vs. Schema on Write
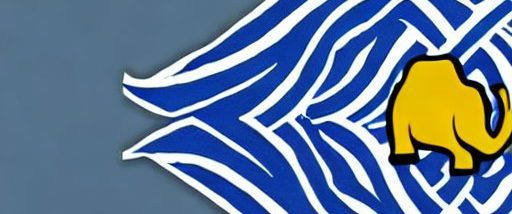
One of the challenges for any Big Data solution is dealing with scale, and RDF stores are no exception: going for billions of RDF triples (the equivalent of rows in the SQL world) is not trivial. Hadoop on the other hand is great at scaling out on commodity hardware, which is a feature every MPP […]
Read More →